Simple Slope
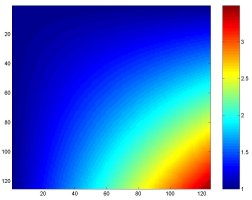
These emulates demonstrate the tradeoffs best.
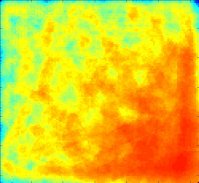
DR = 0.000
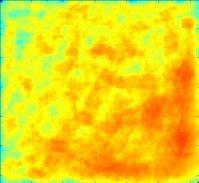
DR = 0.050
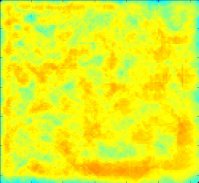
DR = 0.100
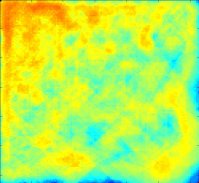
DR = 0.150
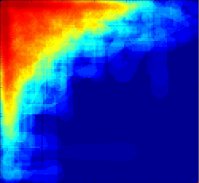
DR = 0.200
Clearly, the best uniform illumination occurs for DR = 0.100. For DR = 0.000, there is no compensation for sensor range variation and we are "looking under the lampost". For DR = 0.200, there is gross overcompensation. The performance for DR = 0.100 comes at the cost of lower performance in other regions. This is because more agent attention is required for difficult search regions.
